Data in agriculture.
“Ugh, this topic is so 2015” you might be thinking. “Just give me some GenAI buzzwords to kick around at my next water cooler chat!”
Well, I’m here to deliver some news. We can’t get to Generative AI or automation or precision agriculture or all the other 2023 hotness without boring, high quality data. So data is where we will begin.
We’re talking about farm data, and more specifically data from the field: where it comes from, how farmers use it today and what the future of data may look like.
Let’s dig in!
Current state of digitization in agriculture
Several years ago when I worked at a farm management software company, I drove out one morning to a Denny’s off I-5 to help a new customer get started with the mobile app. Manny sat down and apologized – he had just been out spreading manure and hadn’t had time to change before our meeting. After the 2 hour drive, I was just grateful he made the time to meet with me.
We got to talking and getting him set up. First, download the app. Did he have his Apple password? I asked. He couldn’t remember it, but he was sure his son would be able to. We called his son, who like any good Millennial kid was his parent’s unofficial IT guy. After 30 minutes on the phone guessing different variations, we finally got the password and the app downloaded.
Another 30 minutes of training, Manny was all set up to collect data on any spraying or spreading activities on his fields. Now, the hard part: Manny would need to remember to open the app and enter data every single time he went out to the field to get a job done.
This is the current state of digitization of agriculture data. It is so rarely the case that digital tools are plug-it-in-and-it-works. It is not seamless. It requires consistent effort and human support.
It doesn’t take long to find stories from farmers of hours spent in the sun dealing with different tools to collect data – tinkering, trial and error, and in many cases throwing up hands in frustration and a “I just need to finish planting. I don’t have time for this!” This friction is one reason that agriculture is among the least digitized industries today.
There are many surveys to understand how much data is being collected by farms today. Across a couple of surveys in the US, the percentage of farmers who collect at least some form of digital data ranges between 80% and 93%, which is relatively high.
The level of digitization – turning data into a format that computers can interpret – is highly variable around the globe. The amount of data collected depends on internet connectivity, level of mechanization, access to data-generating equipment, and the relative value of collecting data.
Digitization requires change. As we will walk through below, as much as digitization unlocks new possibilities, it requires the adoption of technology to collect the data in the first place. Most important to remember, digitization is not the end goal; being able to do new things – or old things in better new ways – is.
Data in → Data Out
While digitization in agriculture is still no walk in the park, there has been a proliferation of digital tools over the past decade to make it easier to collect and use data. To make sense of this landscape, and categorize new technologies and business models, I use a dead simple framework:
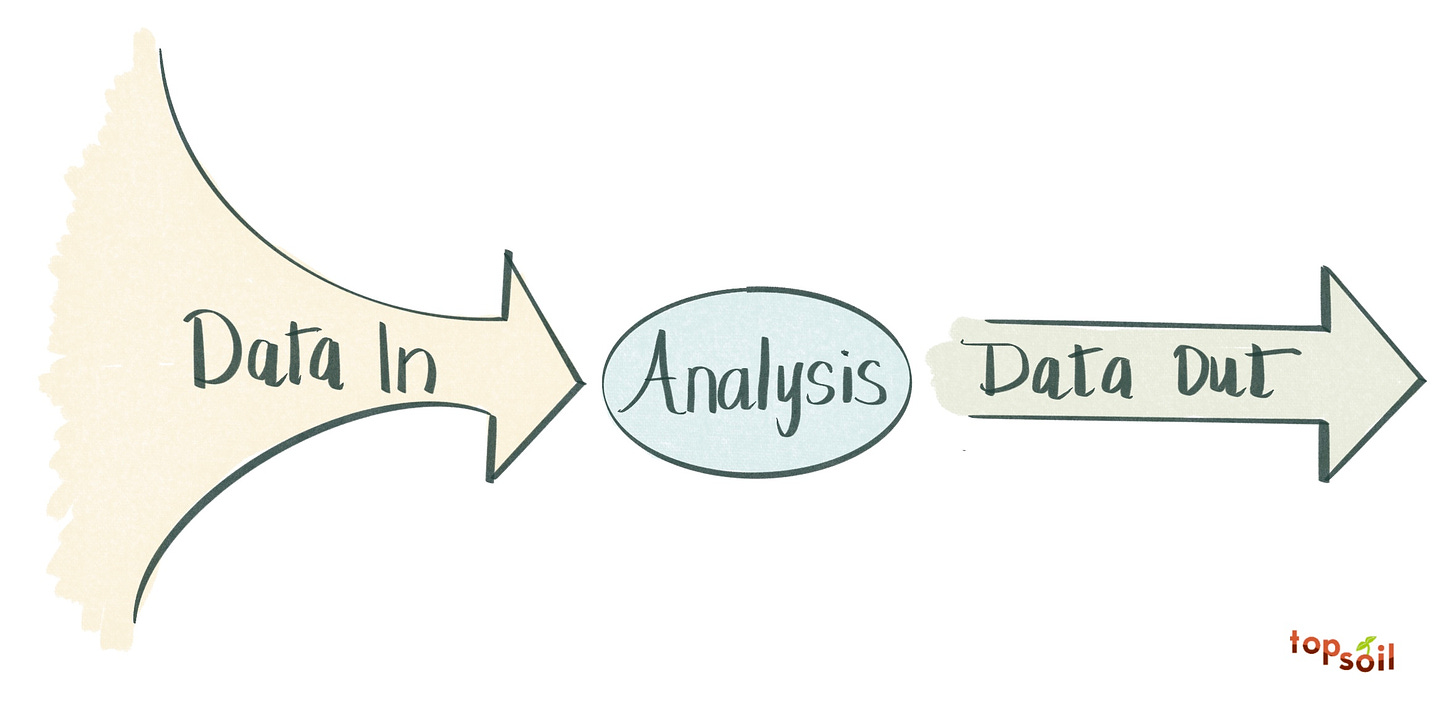
Whenever I read a story about a different kind of sensor or new thing farmers can do with data, it’s helpful to think through the data-in, analysis, and data-out that is being used.
Data In: Where does field data come from?
From the very beginning of agriculture, farmers have been observing what happens in their fields. Data are simply these observations at scale, recorded in a digital format.
There are many sources of data from the field today, with new sensors and types of data being introduced every year:
Beyond these sources of data, there are other types of data that apply to the whole farm, like financial statements, market prices, crop storage data (from grain bins or coolers), or inventory data. While we won’t be talking about this farm-level data today, it’s important to keep in mind that the farm may be collecting data in many different formats for various purposes.
Years ago, one of my business school professors opened class with a hot take: “I hate interesting information.” He was the CEO of a Chicago deep dish pizza restaurant chain, and from his years of running this successful business, he came to the conclusion that if he couldn’t take action with the information he was given, he’d prefer not to know.
Many farmers operate their farm businesses with a similar approach, focusing their data collection in areas where they can take action. I recently spoke with a berry farmer who had just invested in a custom tool to track the individual harvest efficiency of each employee as he explained, “my top three costs are labor, labor, and labor.” As you can imagine, the emphasis of data collection will vary based on the type of crop and geography, among other factors.
Beyond selecting the type of data to collect, spatial and temporal resolution are important factors. A simple non-ag example: if I was signing up for a yoga class, it would be pretty unhelpful if it was advertised as “class takes place in the US” (spatial resolution is not specific enough). If the class was advertised as taking place as “once a year” that might not be satisfactory to reach my yogi goals (temporal resolution is insufficient to be useful).
The key here is that the type of data, the spatial resolution, and temporal resolution have to be right for the planned use of the data. Greater resolution does not always mean more benefit to the farmer – in many cases, greater resolution is more expensive and requires more effort, so farmers must receive incremental value in that additional resolution to justify their investment.
For example, many farmers who practice variable rate seeding or fertility will invest in soil mapping with EC sensors because the publicly available soil maps from SSURGO do not have the level of spatial resolution (or accuracy) to create actionable zones.
There are a handful of common challenges in collecting, storing and using data that get in the way of farmers realizing value from their data:
Connectivity: In many rural agricultural areas, internet connectivity may be spotty at best, which can make it a challenge to upload or download data or run software that requires internet. In a study of farmers in the US, 63% said data network connectivity was a barrier to sharing data.
Interoperability: As my friend Rhishi Pethe (who writes an excellent newsletter Software is Feeding the World) explains, interoperability is “the ability of different agricultural systems and software to exchange, understand, and use data with each other.” Unlike other industries where there is a common data standard, there are several competing data formats in agriculture. When systems don’t talk to each other, it makes it harder to bring together different data sources or to share data.
Quality: There are several dimensions of data quality, but a few that play a large role in agriculture are accuracy (is the data correct?), completeness (are there many missing values?), and consistency (are the values the same between sources?). Farms may be sitting on mountains of data, however, that data may be of low quality because it falls short one or more of these dimensions. This is where we get the phrase, “garbage in, garbage out” – if the quality of the data going in isn’t good, then it makes it much harder for any of the data coming out to be useful.
Trust and Privacy: Farmers must have confidence in how their data is being collected, stored and shared. This means transparency from anyone who touches agriculture data and safeguards to ensure that the data is not used in a way that could harm the farmer. Trust is still a huge barrier for farmers to collect and share their data.
Incentives: In some cases, the person who is responsible for collecting the data is not necessarily the same person that gets value from the data being collected. As an example, an operator who is employed to harvest a crop must make sure the yield monitor is calibrated for accurate data collection. While this creates an extra step for the employee during a busy time, it is the farm owner who will benefit most from the resulting accuracy of the yield maps.
Data Out: How is data used?
From the early 2010’s, farmers have heard that their “data is the new oil.” They were encouraged to tap into this rich new resource and promised that once the data was piped into a software tool, the crude inputs would transform into outputs that would power the future of their farm and the industry as a whole.
But over the past decade, a more apt analogy for agriculture is that data is a new byproduct. Data are the hides, entrails and bits of bone leftover after the more valuable chuck and ribeye are parceled out. It certainly can be valuable, but oftentimes, it often is collected as an afterthought to an activity that needs to get done whether data is being recorded or not. It takes planning, effort and creativity to turn these byproducts (data) into other value streams.
One astute observation from
is that, “in an industry that is really good at creating value from its byproducts, I can't even name one example where a byproduct of a commodity became more valuable than the primary commodity itself.” Data is not inherently valuable on its own, and it is certainly not more valuable than the actual crop being produced.So, how are farmers getting value from their data today?
Decision-making: Like many other industries, farm data is used to improve decision-making. When farmers were asked in two different studies the benefit they experienced from their data, “increased yield” was the number one response. In one of these studies, surveying 800 US Midwestern corn and soybean growers, nutrient management decisions were the most heavily influenced by data (54% stating that these decisions were “influenced by data a lot”).
Beyond decisions to manage nutrients, nearly every other decision throughout the season could potentially be influenced by data. This might mean taking action in-season (for example, scouting a field with satellite imagery and fixing a broken irrigation pivot nozzle) or taking action next season (collecting yield monitor data and analyzing which seed varieties were top performers and selecting those winners to plant the next spring).
Enabling other technologies: Data from the field is used to enable other technologies like precision agriculture and more recently, automation.
Sharing with advisors: In a survey of US farmers, 74% share their data with outside service providers, like their agronomist (58%) or ag input supplier (44%), primarily for agronomic recommendations. Farmers may also share their data with a landowner or banker for access to improved lease and loan terms.
Compliance: In some cases, farmers may share their data with the government for regulatory compliance, or to receive tax incentives, access payment programs, or subsidized insurance.
At the end of the day, farmers go through the effort of collecting, analyzing, and using their data to drive profitability, increase efficiency, improve sustainability, or manage risk.
Where does data go from here?
While data has made an impact in agriculture in some areas, we are still in the early innings in what many consider the “fourth agricultural revolution.”
Based on how the past 30 years have gone, it will continue to get easier and cheaper for data to be collected, stored, securely shared, analyzed, and put to use. We are beginning to see exciting, completely new sources of data (like using plants themselves as sensors). As spatial and temporal resolution improves for sensors and equipment, precision agriculture will manage at a more and more precise granularity – from a field-level to a zone-level (where we are at today) to a plant-level.
Hopefully, these technologies can become more accessible beyond the farmers in the most advanced agriculture economies and be appropriately adapted for unique agricultural systems around the world.
We will likely see the uses for data above improve and expand. As fintech and AgTech collide, farms of the future will get preferential access to resources (or if you’re the glass half-empty type – lose access to resources) like financing, insurance coverage, and land leases based on their data. As there are more and more absentee landowners (at least in the US), you can imagine that some landowners would prefer tenants with strong data practices that can share more information about how the land is used.
Having a strong data strategy may allow farms of the future to access new streams of income. With a more connected ag value chain, premiums that grocery shoppers, like you and I, pay for certain quality factors or growing practices can be allocated back to the farms that have the data to back up their claims. Organic premiums are an example of this that works today, and you can imagine more differentiated categories with greater transparency.
Today, data from the field allows some early adopter farmers to participate in carbon markets to get paid for sequestering carbon in their soils. With an increasing emphasis on environmental sustainability, there will be additional sources of income for farmers who can prove that they are providing other ecosystem services, like supporting biodiversity.
When we peer deeper into the crystal ball many years into the future, other possibilities arise, including improvements in predictions themselves. As modeling becomes more advanced and more data becomes available, the holy grail of predicting the impact of the environment, management techniques, and plant genotype on yield and other outcomes farmers care about becomes more plausible.
But what about AI?
A lot of AI applied to agriculture today (like FBN’s Norm) is focused on the analysis and output of data. In addition to that neat use case (and several other fascinating possibilities that
outlines), I think making it easier to collect data is where AI will have the most immediate impact.So much farm data exists in unstructured notes: paper and pen, a note taking app, back-and-forth texts between the farmer and their crew, phone calls, WhatsApp voice messages, or directions passed through walkie-talkies to name a few.
One of the incredible things that AI (large language models like ChatGPT in particular) is good at is making meaning from unstructured data like rambly notes and transforming it to a format that could be understood and usable elsewhere. Especially in places where we are years or decades away from every piece of equipment automatically recording every application with precision, making data collection easier through AI could be a gamechanger.
Thank you for reading, sharing and subscribing to Topsoil - a monthly newsletter with frameworks to help you make sense of agriculture, at just the right depth.
I sat down with Tim and Tyler from the Modern Acre earlier this month for my favorite conversation together yet, on creating markets in agriculture and how this looks at Nuss Farms. You can check out that podcast episode here!
Topsoil is handcrafted just for you by Ariel Patton. Complete sources can be found here. Thank you to Lauren M. and Andrew M. for generously filling my blind spots. All views expressed and any errors in this newsletter are my own.
How did you feel about this monthly edition of Topsoil? Click below to let me know!
Very interseting outlook! Loved the quote 'he came to the conclusion that if he couldn’t take action with the information he was given, he’d prefer not to know.'.
Often, as tech people, it's hard for us to grasp that people prefer not to know. We grasp data as a gold mine, the more the better.
I was curious to see that none of the answers about data was 'saving time'. I know that agritculture is very time intensive, especially during the summer. Having accurate and relevant data can 'free' the farmers from crazy work days and give them some room to be with the families, or even more free time to dedicate to other important decisions.